Over the past decade, we have seen quite a lot of discrepancies between the marks on an OSCE scoresheet and the Global Rating Scale (GRS) with the feedback provided. The result is that students seem to fail looking at their scores, but pass or perform even better on their GRS according to their examiners.
How come?
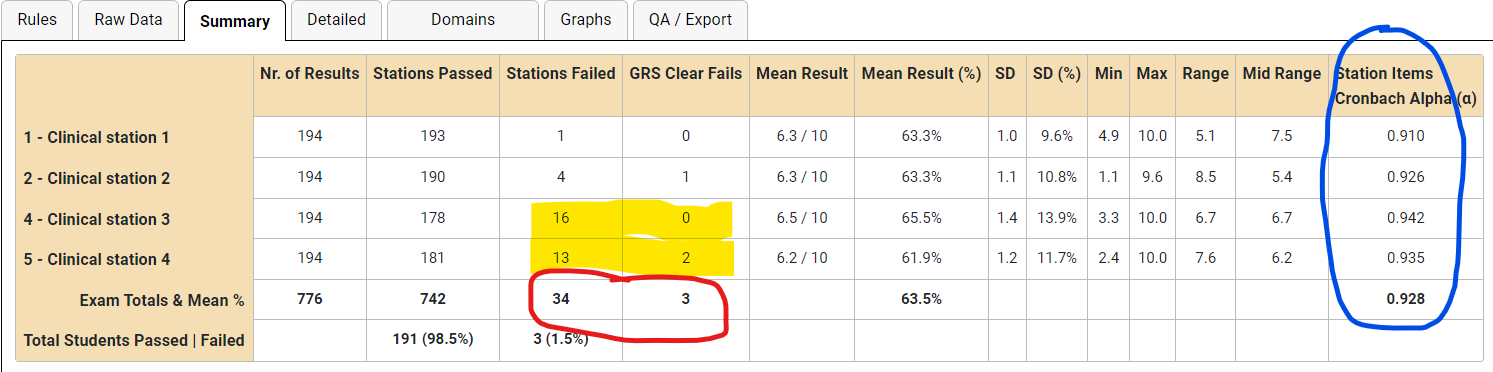
In a recent example from a year 5 exam, we can see that 34 students failed (column 3) their exam based on their item scoresheet, whereas only 3 failed on the GRS (column 4). Although the internal consistency of the item scoresheet is high (Cronbach’s Alpha > 0.9), examiners marked students’ performance as insufficient according to the item scoresheet although only 3 students failed according to the GRS.
We see this inconsistency amongst many clients using Qpercom’s bespoke OSCE Management Information System. One of the reasons for this inconsistency might be due to the fact that in the default setting of the performance scoresheet, the examiners mark the students ‘blind’, on a Likert scale from 0 to 4. In general, ‘0’ stands for ‘not done’ and ‘4’ stands for ‘done and complete’, while observing and marking a specific clinical skill. All ‘done and complete’ scores should result in an Excellent score on the GRS, whereas ‘not done’ scores should result in a Fail or at most a Borderline. Other possible scores are Pass and Good.
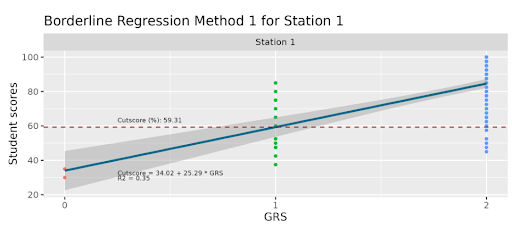
What we often see is similar to what you see in the image above, a chart addressing a three item Likert scale with 0 = Fail, 1 = Borderline and 2 = Pass. If we look at the scores (in blue) for Pass, they range from way below the (adjusted) cut score of 59.31 up to the maximum score possible (100). Even the range of scores around Borderline vary from less than 40 to 100. As you can see, the explaining variance in this regression curve is only 35% which is considered poor, and much ‘error’ is observed around the curve. Only two of the ‘Fail’ scores (0) on the X-axis match with the scores on the Y-axis, and quite a few don’t match with Borderline and Pass ratings.
The written feedback added to the scores and GRS(examiners’ overall impression of a student’s performance) often doesn’t offer anything but confusion for the student receiving these kinds of results. We’re currently awaiting the outcome of a PhD study analysing whether written feedback matches either the scores and/or the GRS of the examiner assessing the students.
Enthusiastic about the arrival of ChatGPT which surfaced around November 2022, we have experimented with adding item scores, global rating scores and written feedback into ChatGPT to see what we would get back from this Artificial Intelligence language model chatbot.
For the highest performing student, ‘Mark’, this is what the chatbot came back with (500 words only):
[/et_pb_text][et_pb_text _builder_version=”4.4.0″ text_font=”Cabin||||||||” text_font_size=”16px” background_size=”initial” background_position=”top_left” background_repeat=”repeat” width=”100%” hover_enabled=”0″]
“Mark performed very well in his year 5 clinical skills exams. In Clinical station 1, he scored 90.0%, in Clinical station 2, he scored 80.0%, in Clinical station 3, he scored 100.0%, and in Clinical station 4, he scored 86.0%. These scores indicate that Mark has a strong understanding of clinical skills and is able to apply them effectively.
In the patient-related stations, which focus on physical examination, Mark scored 3, 2.4, 3, and 2.4 out of 5, respectively. In terms of his ability to interpret physical signs and provide a differential diagnosis, Mark scored 2.4, 2.4, 3, and 3 out of 3. These scores suggest that Mark has a good understanding of physical examination techniques and is able to apply them in the diagnosis of patients.
For case discussion, Mark scored 2, 1.6, 2, and 1.6 out of 3. These scores indicate that Mark is able to discuss cases effectively and provide appropriate management plans.
In terms of professionalism, communication, and maintaining patient safety, Mark scored 1.6, 2, 1.6, and 1.6 out of 3. These scores suggest that Mark has a good understanding of the importance of professionalism, communication, and patient safety, but there may be room for improvement in these areas.
Overall, Mark’s performance in the exams was rated as “Excellent” by the examiners. One of the examiners provided positive written feedback, describing Mark as a “really strong candidate” who is “very confident and appropriate” and “progressed very well”. The examiner also noted Mark’s “excellent differential and management plan”.
In conclusion, Mark has demonstrated a strong understanding of clinical skills and is able to apply them effectively in the diagnosis and management of patients. While there may be room for improvement in areas such as professionalism, communication, and patient safety, Mark’s overall performance was rated as “Excellent” and he received positive feedback from one of the examiners”.
Providing ChatGPT with slightly more information about the GRS, it came back with the following feedback for the lowest performing student, ‘Lea’:
“Based on the scores provided, Lea’s performance in the clinical skills exams is below average, with scores ranging from 23% to 51%. It is important to note that the scores are not the only indicator of performance, but they are a significant factor in evaluating a student’s ability to apply clinical skills.
Looking at the patient-related stations, Lea’s ability to approach and identify physical signs scored below average, with scores ranging from 50% to 55%. Additionally, Lea’s ability to interpret and provide differential diagnosis of physical signs scored slightly better, but still below average, with scores ranging from 45% to 50%. Case discussion and Professionalism scores are also below average, with scores ranging from 30% to 37%.
Given the above scores, it is likely that Lea will receive a fail or borderline result. It is important to note that there may be mitigating factors that could have affected Lea’s performance, such as test anxiety, poor time management, or inadequate preparation. It may be helpful to discuss these factors with Lea to identify any areas for improvement.
In terms of feedback, it would be helpful to provide specific recommendations for improvement in each area where Lea scored below average (as provided by some of the examiners). For example, in the patient-related stations, Lea may benefit from additional training in physical examination techniques or differential diagnosis. In the case discussion and professionalism areas, Lea may benefit from additional training in communication skills, patient safety, or ethical considerations.
Overall, it is important to emphasise that these scores do not define Lea’s potential as a medical student or future physician. With dedication, hard work, and targeted training, Lea can improve and ultimately excel in the clinical skills required for a successful career in medicine.”
Due to this finding (and other internal experiments) it is most certainly worthwhile to consider matching the ChatGPT Application Programming Interface (API) with the Qpercom Observe (API). Extended research is required regarding data storage and integrity using openAI. The more detail we provide to the chatbot API, the more precise and consistent the internal feedback will be, adding to the (small) details sometimes provided by generally very inconsistent examiners.